In the fast-paced world of artificial intelligence and machine learning, hardware innovations play a pivotal role in driving progress and enabling breakthroughs in various industries. Google, a leading tech giant, has been at the forefront of such innovation with its development of the Tensor Processing Unit (TPU), a specialized chip designed to accelerate AI workloads. In this blog, we’ll delve into the world of Google’s Tensor Chip, exploring its significance, impact, and the future it promises.
The Genesis of Google’s Tensor Chip
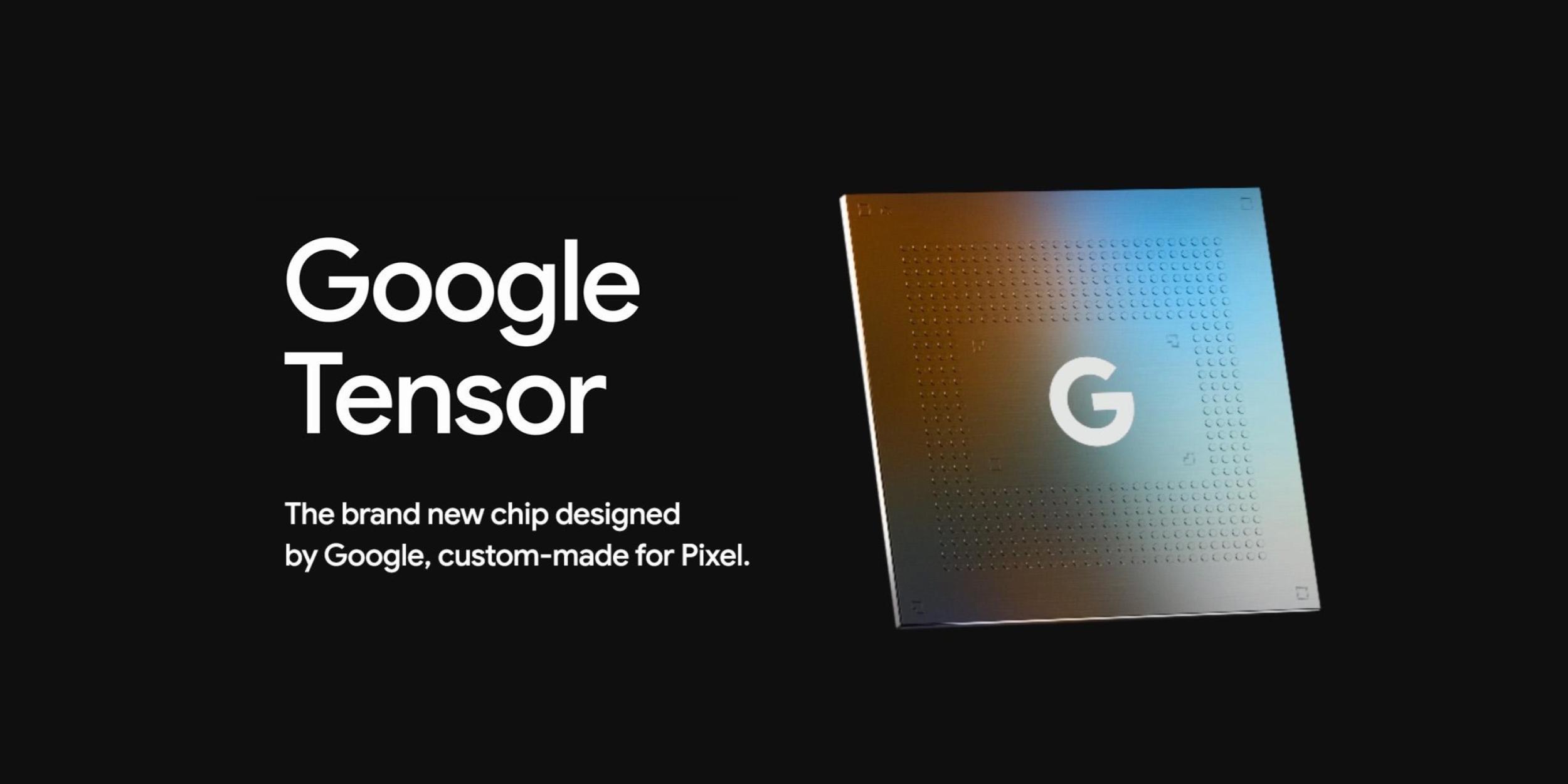
The concept of specialized hardware for AI and machine learning tasks has been around for quite some time. Graphics Processing Units (GPUs) from companies like NVIDIA have been repurposed for these tasks due to their parallel processing capabilities. However, these GPUs, although highly effective, were not designed from the ground up to handle AI workloads. Google recognized the need for dedicated hardware and set out to create the Tensor Processing Unit.
1. The first iteration of TPU
It is known as TPUv1, was introduced in 2015 and was a crucial step in Google’s journey to revolutionize AI hardware. It was primarily designed for inference tasks, enabling models to make predictions quickly and efficiently. However, it was with the release of TPUv2 in 2017 that Google made a more significant impact.
2. Tensor Processing Unit 2.0 (TPUv2)
This brought improvements in both training and inference capabilities. Its high-performance capabilities caught the attention of AI researchers and developers. Google was able to harness TPUs in its data centers, providing cloud-based access to this powerful hardware for anyone who needed it.
3. Tensor Processing Unit 3.0 (TPUv3)
It continued the trend with even more improvements in performance and scalability. And, as we’ll see, Google’s latest TPU iterations have only pushed the boundaries further.
4. Tensor Processing Unit 4.0: The Latest Advancement
The most recent development in this line is the TPUv4, which represents Google’s relentless pursuit of AI hardware excellence. TPUv4 offers remarkable advancements in terms of speed, efficiency, and versatility. With 20 times the performance of TPUv3, TPUv4 can handle the most complex AI and machine learning models with ease. It has an impressive 100 teraflops of computing power and can scale to thousands of TPUs for massive parallel processing.
Essential power for training state-of-the-art models in NLP, computer vision, and reinforcement learning across diverse domains. TPUv4 sets a new AI hardware benchmark, reducing time and cost for training models driving industry innovations.
The Impact of Google’s Tensor Chip
- Accelerating AI Research: Google’s Tensor Chip has been a game-changer for the AI research community. Accelerates research with larger, complex models, reducing training time and enabling faster iterations for researchers. This acceleration has led to numerous breakthroughs in AI, from more advanced language models to improved computer vision systems.
- Democratizing AI: By offering access to TPUs in the cloud, Google has democratized AI. It has allowed smaller companies and individual developers to harness the power of TPUs without the need for expensive hardware investments. This accessibility has fostered a vibrant ecosystem of AI development and innovation.
- Advancements in Deep Learning: Deep learning models, particularly neural networks with numerous layers, require substantial computational power for training. Google’s Tensor Chip enables efficient deep learning model training, driving progress in speech recognition, autonomous vehicles, and medical image analysis.
- Enhanced Natural Language Processing: Language models like BERT and GPT-3 have transformed natural language processing. Google’s Tensor Chip has played a pivotal role in training these models, making them faster and more accessible. This has implications for improving chatbots, machine translation, sentiment analysis, and more.
- Optimizing Recommendation Systems: Recommendation systems used by companies like Netflix and Amazon rely on AI models to personalize content for users. TPUs enhance recommendation systems, improving user experiences through efficient processing of extensive user data.
The Future of AI with Google’s Tensor Chip
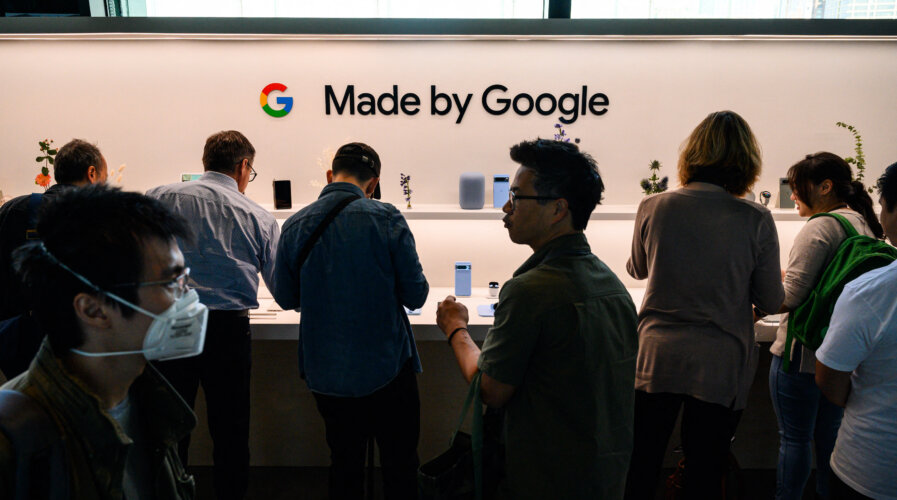
With advancing tech, Google’s Tensor Chip leads the way in shaping the future of AI and machine learning. Here are some of the potential developments and impacts we can anticipate:
- More Advanced AI Models: With the increasing computational power of TPUs, we can expect the emergence of even more advanced AI models. These models will be capable of solving complex problems and providing innovative solutions in areas like healthcare, finance, and climate science.
- Real-time AI Applications: The speed and efficiency of Google’s Tensor Chip can enable real-time AI applications, such as instant language translation, autonomous vehicles that can react to complex traffic situations in milliseconds, and medical diagnostics with immediate results.
- Edge AI: The miniaturization of TPUs and their energy-efficient design will enable the deployment of AI at the edge, closer to where data is generated. This will lead to faster, more responsive AI systems in IoT devices, smartphones, and other edge computing environments.
- Advancements in Robotics: The combination of AI and robotics is poised to make significant strides. Robots equipped with TPUs will be capable of complex decision-making, improving automation in industries like manufacturing, agriculture, and healthcare.
- Ethical AI and Bias Mitigation: AI integration highlights the growing importance of addressing ethics and bias mitigation in AI systems. Google’s Tensor Chip can play a role in developing more robust and unbiased AI models.
Conclusion
Google’s Tensor Chip has had a profound impact on the field of artificial intelligence and machine learning. It has accelerated AI research, democratized access to powerful AI hardware, and enabled advancements in deep learning, natural language processing, and recommendation systems. Future AI developments: advanced models, real-time apps, edge AI, robotics, and ethical AI emphasis as technology evolves. Google’s Tensor Chip is paving the Way for Transformative AI Applications.
Leave a Reply